This is an early access version, the complete PDF, HTML, and XML versions will be available soon.
Open AccessArticle
MVG-Net: LiDAR Point Cloud Semantic Segmentation Network Integrating Multi-View Images
by
Yongchang Liu
,Yawen Liu
andYansong Duan
*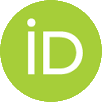
School of Remote Sensing and Information Engineering, Wuhan University, Wuhan 430079, China
*
Author to whom correspondence should be addressed.
Remote Sens. 2024, 16(15), 2821; https://doi.org/10.3390/rs16152821 (registering DOI)
Submission received: 31 May 2024 / Revised: 29 July 2024 / Accepted: 30 July 2024 / Published: 31 July 2024
Abstract
Deep learning techniques are increasingly applied to point cloud semantic segmentation, where single-modal point cloud often suffers from accuracy-limiting confusion phenomena. Moreover, some networks with image and LiDAR data lack an efficient fusion mechanism, and the occlusion of images may do harm to the segmentation accuracy of a point cloud. To overcome the above issues, we propose the integration of multi-modal data to enhance network performance, addressing the shortcomings of existing feature-fusion strategies that neglect crucial information and struggle with matching modal features effectively. This paper introduces the Multi-View Guided Point Cloud Semantic Segmentation Model (MVG-Net), which extracts multi-scale and multi-level features and contextual data from urban aerial images and LiDAR, and then employs a multi-view image feature-aggregation module to capture highly correlated texture information with the spatial and channel attentions of point-wise image features. Additionally, it incorporates a fusion module that uses image features to instruct point cloud features for stressing key information. We present a new dataset, WK2020, which combines multi-view oblique aerial images with LiDAR point cloud to validate segmentation efficacy. Our method demonstrates superior performance, especially in building segmentation, achieving an F1 score of 94.6% on the Vaihingen Dataset—the highest among the methods evaluated. Furthermore, MVG-Net surpasses other networks tested on the WK2020 Dataset. Compared to backbone network for single point modality, our model achieves overall accuracy improvement of 5.08%, average F1 score advancement of 6.87%, and mean Intersection over Union (mIoU) betterment of 7.9%.